<img src="https://raw.githubusercontent.com/chenzhaiyu/abspy/main/docs/source/_static/images/logo.png" width="480"/>
-----------
[](https://opensource.org/licenses/MIT) [](https://pypi.python.org/pypi/abspy/) [](https://abspy.readthedocs.io/en/latest/)
## Introduction
***abspy*** is a Python tool for 3D *adaptive binary space partitioning* and beyond: an ambient 3D space is adaptively partitioned to form a linear cell complex with pre-detected planar primitives in a point cloud, where an adjacency graph is dynamically obtained. Though the tool is designed primarily to support compact surface reconstruction, it can be extrapolated to other applications as well.
## Key features
* Manipulation of planar primitives detected from point clouds
* Linear cell complex creation with adaptive binary space partitioning (a-BSP)
* Dynamic BSP-tree ([NetworkX](https://networkx.org/) graph) updated locally upon insertion of primitives
* Support of polygonal surface reconstruction from graph cuts
* Compatible data structure with [Easy3D](https://github.com/LiangliangNan/Easy3D) on point clouds, primitives, cell complexes and surfaces
* Robust Boolean spatial operations underpinned by the rational ring from [SageMath](https://www.sagemath.org/)'s exact kernel
## Installation
### Install requirements
All dependencies except for [SageMath](https://www.sagemath.org/) can be easily installed with [PyPI](https://pypi.org/):
```bash
git clone https://github.com/chenzhaiyu/abspy && cd abspy
pip install -r requirements.txt
```
Optionally, install [pyglet](https://github.com/pyglet/pyglet) and [pyembree](https://github.com/adam-grant-hendry/pyembree) for better visualisation and ray-tracing, respectively:
```bash
pip install pyglet pyembree
```
### Install SageMath
For Linux and macOS users, the easiest is to install from [conda-forge](https://conda-forge.org/):
```bash
conda config --add channels conda-forge
conda install sage
```
Alternatively, you can use [mamba](https://github.com/mamba-org/mamba) for faster parsing and package installation:
```bash
conda install mamba
mamba install sage
```
For Windows users, you may have to build SageMath from source or install all other dependencies into a [pre-built SageMath environment](https://doc.sagemath.org/html/en/installation/binary.html).
### Install abspy
***abspy*** itself can be found and easily installed via [PyPI](https://pypi.org/project/abspy/):
```bash
pip install abspy
```
## Quick start
Here is an example of loading a point cloud in `VertexGroup` (`.vg`), partitioning the ambient space into candidate convexes, creating the adjacency graph, and extracting the outer surface of the object.
```python
import numpy as np
from abspy import VertexGroup, AdjacencyGraph, CellComplex
# load a point cloud in VertexGroup
vertex_group = VertexGroup(filepath='points.vg')
# normalise the point cloud
vertex_group.normalise_to_centroid_and_scale()
# additional planes to append (e.g., the bounding planes)
additional_planes = [[0, 0, 1, -bounds[:, 0, 2].min()]]
# initialise CellComplex from planar primitives
cell_complex = CellComplex(vertex_group.planes, vertex_group.bounds, vertex_group.points_grouped, build_graph=True, additional_planes=additional_planes)
# refine planar primitives
cell_complex.refine_planes()
# prioritise certain planes (e.g., vertical ones)
cell_complex.prioritise_planes(prioritise_verticals=True)
# construct CellComplex
cell_complex.construct()
# print info on the cell complex
cell_complex.print_info()
# build adjacency graph of the cell complex
adjacency_graph = AdjacencyGraph(cell_complex.graph)
# assign weights (e.g., SDF values provided by neural network prediction) to graph
weights_dict = adjacency_graph.to_dict(...)
adjacency_graph.assign_weights_to_n_links(cell_complex.cells, attribute='area_overlap', factor=0.001, cache_interfaces=True)
adjacency_graph.assign_weights_to_st_links(weights_dict)
# perform graph-cut to extract surface
_, _ = adjacency_graph.cut()
# save surface model to an OBJ file
adjacency_graph.save_surface_obj('surface.obj', engine='rendering')
```
Usage can be found at [API reference](https://abspy.readthedocs.io/en/latest/api.html). For the data structure of a `.vg`/`.bvg` file, please refer to [VertexGroup](https://abspy.readthedocs.io/en/latest/vertexgroup.html).
## Misc
* **Why adaptive?**
Adaptive space partitioning can significantly reduce computations for cell complex creation, compared to the exhaustive counterpart. The excessive number of cells from the latter not only hinders computation but also inclines to defective surfaces on subtle structures where inaccurate labels are more likely to be assigned.
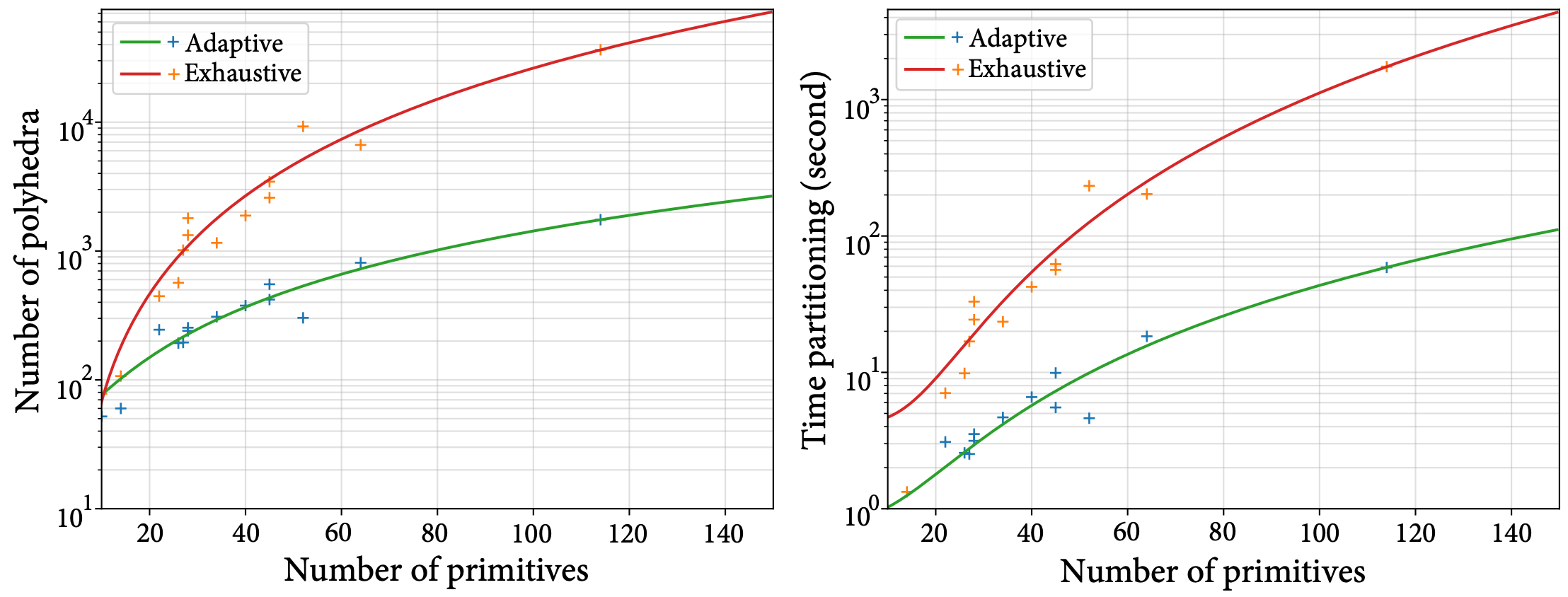
Run the benchmark on the number of candidate cells and runtime among adaptive partitioning, exhaustive partitioning, and SageMath's [hyperplane arrangements](https://doc.sagemath.org/html/en/reference/discrete_geometry/sage/geometry/hyperplane_arrangement/arrangement.html):
```bash
python misc/benchmark.py
```
* **How can abspy be used for surface reconstruction?**
With the cell complex constructed and its adjacency maintained, surface reconstruction can be addressed by a graph cut solver that classifies each cell as being *inside* or *outside* the object. The surface exists in between adjacent cells where one is *inside* and the other is *outside* — exactly where the cut is performed. For more information, refer to [Points2Poly](https://github.com/chenzhaiyu/points2poly) that wraps ***abspy*** for building surface reconstruction.
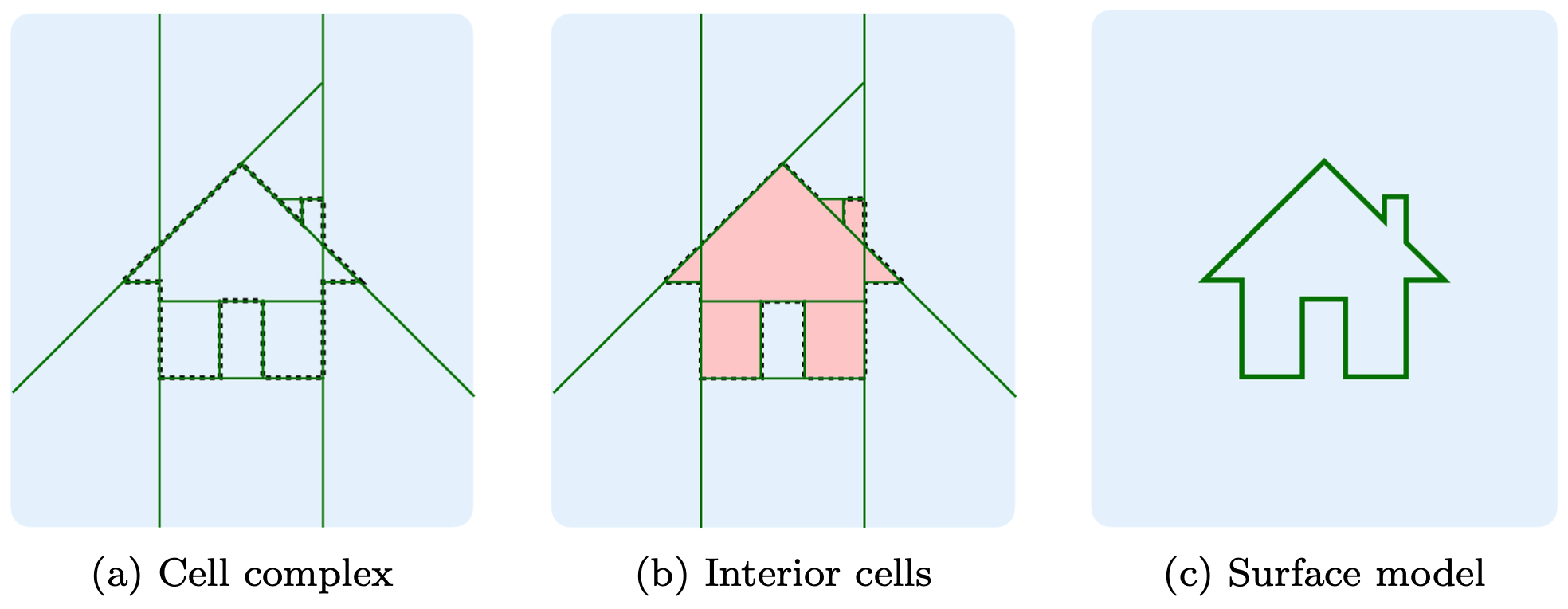
## License
[MIT](https://raw.githubusercontent.com/chenzhaiyu/abspy/main/LICENSE)
## Citation
If you use ***abspy*** in a scientific work, please consider citing the paper:
```bibtex
@article{chen2022points2poly,
title = {Reconstructing compact building models from point clouds using deep implicit fields},
journal = {ISPRS Journal of Photogrammetry and Remote Sensing},
volume = {194},
pages = {58-73},
year = {2022},
issn = {0924-2716},
doi = {https://doi.org/10.1016/j.isprsjprs.2022.09.017},
url = {https://www.sciencedirect.com/science/article/pii/S0924271622002611},
author = {Zhaiyu Chen and Hugo Ledoux and Seyran Khademi and Liangliang Nan}
}
```